Devers vs. Cole: A Statistically Improbable Matchup
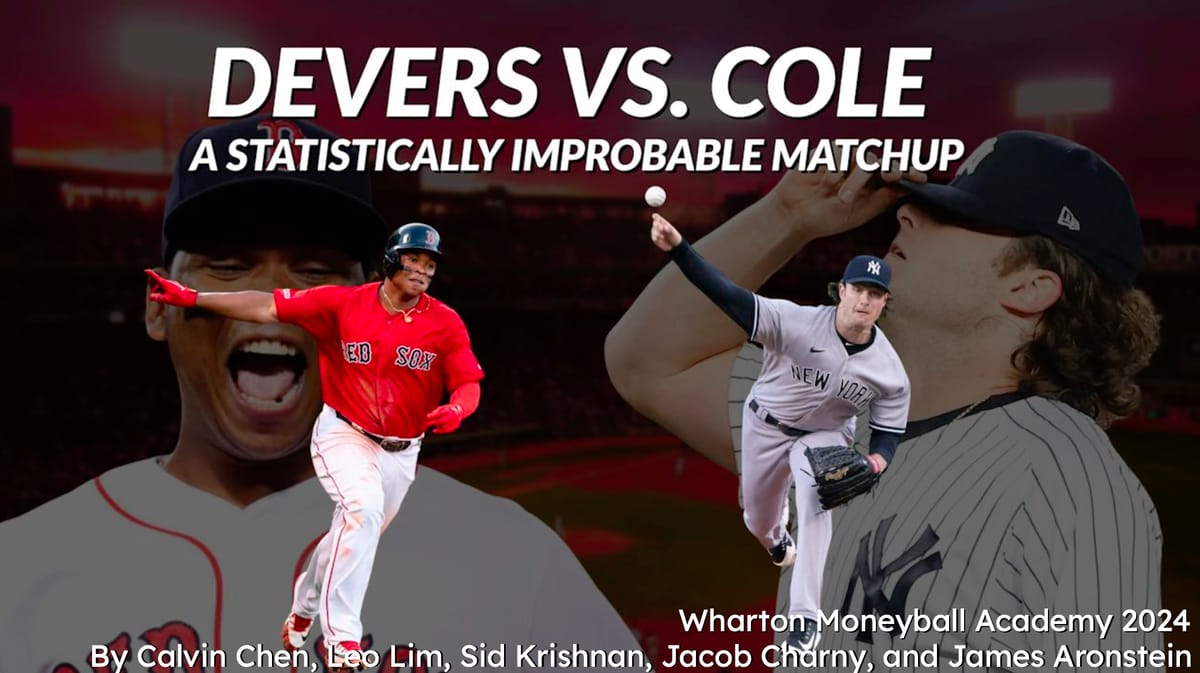
On June 7th, 2024, during the intense rivalry game between the Boston Red Sox and New York Yankees at Yankee Stadium, Rafael Devers hit a 441-foot home run to center field against Gerrit Cole, an extraordinary pitcher who just came back from rehab after his elbow injury. Despite the magnitude of the home run Devers hit against Cole, users on social media were not surprised: On the MLB’s Instagram post, users commented “Devers once again owns Cole”, “Devers telling who Cole’s father is once again”, and “Devers cannot stop raking against Cole”. These mundane reactions to Devers’s dinger against one of the best pitchers in the MLB in the 2020s can be justified by the insane individual stats Devers has particularly on Gerrit Cole. Wondering if this unbelievable performance is due to Devers’s luck or his pure skill that counteracts Gerrit Cole, I decided to conduct a research to investigate this improbable matchup between the two players.
First, before going into the specifics, I researched the stats for Rafael Devers and Gerrit Cole in their respective career. Bursting onto the scene in 2017, Rafael Devers has been a solid power hitter with good contact: He since has collected 1012 hits, 195 of which being home runs. Also, he has a slash line of .281/.346/.516, indicating that he is a proficient hitter. Due to his impressive stats and his popularity, he has been an All-Star candidate almost every year in his career. Gerrit Cole, beginning in 2013, has been a magnificent pitcher, dominating numerous hitters throughout his career: With a 146W-76L record, he has a 3.19 ERA, collecting almost 2200 strikeouts through 1888 innings. Thanks to his outstanding pitching stats, he has been an All-Star candidate most of his career and even earned a Cy Young Award last season.
Looking at the two players’ career stat lines respectively, we can easily acknowledge that both are wonderful players who dominate the league; However, when it comes to the career stats of Devers against Cole, things get overtly different. Through 15 games and 48 plate appearances, Devers has recorded 14 hits, 9 of which are extra-base hits (XBH); among those XBHs, 8 of them were home runs, collecting 19 RBIs in total. The slash line seems more impressive: .341/.438/.951, and a 1.389 OPS. Considering that Gerrit Cole’s career XBA and XSLG are .223 and .372, respectively, this shows that Devers’ career stats against Cole are an obvious outlier compared to the matchups Gerrit Cole has had in his career.
After looking at the apparent stat line, the comparison was drawn of Rafael Devers with other match-ups, and the graphs corroborated the conclusion made previously.
For advanced statistics that relate to OPS, I selected Hard Hit %, Barrel %, Isolated Slugging %, and Whiff %. Looking at those graphs, it can be easily found that Devers is located on the far right side of the histogram of the respective stats for individual matchups against Cole among the hitters who faced him more than 5 times; In other words, Devers is excelling far compared to other hitters who have faced Gerrit Cole. One interesting fact to note is that on the “Whiff % Against Gerrit Cole” histogram, different from what the other three graphs showed, Devers (red line) is located approximately in the middle among all hitters with more than 5 plate appearances against Cole. This shows that even though maintaining extremely high OPS and OBP against Gerrit Cole, Rafael Devers does swing and misses pitches from Cole, indicating Devers’s plate discipline is not one of the biggest confounding factors to Devers’s unbelievable matchup stats against Cole.
After closely analyzing the data that offers some insight into Rafael Devers's success against Gerrit Cole, I decided to conduct a permutation test on Devers's OPS. The goal was to determine whether his impressive performance was mainly due to skill or just luck. For this, I established a null hypothesis suggesting that Devers's success against Cole is unsustainable and largely driven by luck. Conversely, the alternative hypothesis proposed that Devers's success is rooted in skill and is therefore highly sustainable. To set up the permutation test, I selected left-handed hitters who have more than 502 total plate appearances against righties since 2013 (when the righty pitcher Gerrit Cole came into the league). To be more precise, I selected the players in the sample size to be in the 95th percentile of the OPS against rightie (.857) and have more than 5 plate appearances against Gerrit Cole. After running the permutation test 100,000 times with the programming tool “R”, the mean OPS showed .805 with a standard deviation of 0.218. On the contrary, Devers, with an OPS of 1.389, had a Z-Score of 2.679, lying on the 99.9925th percentile on the distribution of OPS values from the permutation test, giving a P-Value of 0.0075, or 0.75%. This P-value means that there is only a 0.75% probability that the null hypothesis “Devers’ success against Cole is unsustainable, and largely due to luck” is true, leading us to safely reject the null hypothesis and conclude that the statistics Devers has against Gerrit Cole in his career are purely attributable to his sheer skill.
Now, why is Rafael Devers exceptionally strong and successful in the matchups against Gerrit Cole? To determine the factors behind Devers’ success, I compared Devers’ predilection for Gerrit Cole’s pitch types. Below is the bar graph of the frequency of pitches thrown by Cole to Devers based on the pitch types:
According to this graph, Cole has thrown four-seam fastballs the most (102), followed by knuckle curves (43) and changeups (37). Despite his high usage of fastball, consisting of 50.2% of the total pitches he had thrown to Devers, its effectiveness is quite poor, as Devers has .316/.409/.947 against his fastball. Among his 6 hits against Cole’s fastballs, 4 of them were home runs, and the average exit velocity was 103.4% and generating hard hits 88.9%. This is quite counterintuitive as Gerrit Cole’s four-seam fastball has the lowest run value (-15) among all the current pitchers in the MLB, with the opponent's batting average of 0.187, slugging percentage of 0.321, and the K rate of 30.0%. However, this facade contradiction can easily be understood when looking at Rafael Devers’ fun values against fastballs by movement and velocity:
On the left side of the graph, which is the heat map of lefty hitters’(Where Devers is at) run values against RHP fastballs (Where Cole is at), Gerrit Cole has a fastball with a vertical movement of 11 inches and horizontal movement of 12.5 inches and velocity between 95mph and 96.5mph (yellow bin). This bin shows a run value of far less than -10, determined by the color of the bin. This graph indicates that Gerrit Cole has an outstanding value fastball that is one of the best in the league. However, the interesting part is that Rafael Devers has a particularly high run value of over 0.5 on the fastball with (velocity, movement) of (95.5, 11.5), which includes the range of Gerrit Cole’s fastball. Thus, this finding shows that even though Gerrit Cole does have a virtually untouchable four-seam fastball, Rafael Devers’ relatively high run values against the specific pitch constituents of Gerrit Cole’s fastball make it relatively favorable for Devers to reach success against Cole’s fastball, often creating game-changing hits and RBIs.
Similar to Gerrit Cole’s fastball, his changeup is also often demolished by Rafael Devers, because of a similar reason to what is explained above, but in a more obvious manner:
This heatmap demonstrates the run value for spin rate (RPM) for the x variable and movement (in) for the y variable; Gerrit Cole, with a spin rate of about 1807 and vertical-horizontal movement of 14-15.5 inches, has a run value of approximately +2, as illustrated by the yellow bin. On the contrary, Rafael Devers also creates a run value of approximately +1 to +2 for the pitches that have a spin rate of about 1807 and vertical-horizontal movement of 14-15.5 inches. Since the pitches with these traits described are changeups, it can be safely assumed that Rafael Devers is particularly successful in Gerrit Cole’s changeup because of his changeups’ distinctive movement and spring rate, which Devers favors. In addition, Cole’s career changeup run-value is -6, while Devers’ run-value against changeup in his career is +6, which leads to the conclusion that Devers can successfully counteract Cole’s changeup.
Then, what should Gerrit Cole do when he faces Rafael Devers in the future?
Let’s first go back to the graph mentioned earlier:
According to the top graph (black), Cole has thrown four-seam fastballs the most (102), followed by knuckle curves (43) and changeups (37). Accordingly, the total bases created by Devers from Cole’s fastballs is 18, and that created from changeups is 15. However, there is an interesting fact that I want to point out: Despite its second-most usage (43 pitches), there are 0 total bases created by Devers against Cole’s knuckle curves. In addition, against Cole’s knuckle curves, Devers has a slash line of mere .00/.17/.00, meaning he has only reached base once out of 43 pitches. This data leads to the conclusion that if Gerrit Cole throws knuckle curves more frequently, there is a slighter chance for Devers to keep his pace when he faces Cole.
Lastly, I investigated whether Devers’ performance against Gerrit Cole would regress to the mean as they face each other more. To determine this, I created a chart of the historical data of the OPS before and after the 48-plate appearance mark, including the previous matchups of hitters and pitchers who are considered to be outstanding in the league, which is shown below:
According to the graph, the OPS values from the previous matchups in the MLB don't significantly regress toward the mean value after the 48 PA mark. Instead, they tend to have a slope that does not digress far from 0, indicating that whatever OPS level the player-pitcher matchups have settled into after 48 PAs remains relatively consistent, without much regression. In simpler terms, the graph suggests that these matchups, once they hit the 48 PA mark, stabilize at their current performance levels rather than regressing toward a broader average, challenging the typical expectation of regression to the mean in baseball performance stats. Thus, based on the historical matchups, Devers’ performance against Cole will be consistent and sustainable; and he will keep showcasing his ability to consistently perform well against one of the league’s top pitchers.
In conclusion, Rafael Devers' extraordinary success against Gerrit Cole is far from a statistical anomaly; it's a testament to Devers' skill in exploiting specific pitch characteristics that Cole traditionally excels with, which can be successfully backed up by the result and the extremely low P-Value from the permutation test. In addition, the analysis reveals that Devers' performance against Cole remains consistent, even as plate appearances accumulate, challenging the typical expectation of regression to the mean in baseball. This consistency suggests that Devers has a unique ability to counteract Cole's pitching, particularly his fastball and changeup, making him a persistent threat in their matchups. For Cole, this underscores the need for strategic adjustments, such as relying more on his knuckle curve, to mitigate Devers' impact in future encounters.
Code Used for This Project
Permutation Test
library('Lahman')
library('tidyverse')
# Load the data
data <- read_csv('data/Untitled spreadsheet - Sheet1.csv')
# Check the data
print(data)
# Calculate total counts for each type of outcome
total_HR <- sum(data$HR, na.rm = TRUE)
total_3B <- sum(data$'3B', na.rm = TRUE)
total_2B <- sum(data$'2B', na.rm = TRUE)
total_1B <- sum(data$'1B', na.rm = TRUE)
total_BB <- sum(data$BB, na.rm = TRUE)
total_SO <- sum(data$SO, na.rm = TRUE)
total_O <- sum(data$PA, na.rm = TRUE)-
sum(data$SO, na.rm = TRUE)-
sum(data$BB, na.rm = TRUE)-
sum(data$'1B', na.rm = TRUE)-
sum(data$'2B', na.rm = TRUE)-
sum(data$'3B', na.rm = TRUE)-
sum(data$HR, na.rm = TRUE)
total_O
# Construct outcomes vector
outcomes <- c(rep("HR", total_HR),
rep("3B", total_3B),
rep("2B", total_2B),
rep("1B", total_1B),
rep("BB", total_BB),
rep("SRIKEOUT", total_SO),
rep("OUT", total_O))
length(outcomes)
# Parameters for the permutation test
length(sample)
num_samples <- 100000
sample_size <- 48
observed_average_ops <- 1.389
# Run the permutation test
sample_means <- numeric(num_samples)
# Function to calculate OPS
calculate_ops <- function(sample) {
# Calculate plate appearances (PA) and at-bats (AB)
plate_appearances <- length(sample)
at_bats <- sum(sample != "BB")
# OBP calculation
hits <- sum(sample %in% c("HR", "3B", "2B", "1B"))
walks <- sum(sample == "BB")
obp <- (hits + walks) / plate_appearances
# SLG calculation (excluding walks)
total_bases <- sum(sample == "HR") * 4 +
sum(sample == "3B") * 3 +
sum(sample == "2B") * 2 +
sum(sample == "1B") * 1
slg <- total_bases / at_bats
# OPS calculation
ops <- obp + slg
return(ops)
}
# Perform permutation test
for (i in 1:num_samples) {
sample <- sample(outcomes, sample_size, replace = TRUE)
sample_mean <- calculate_ops(sample)
sample_means[i] <- sample_mean
}
# Calculate p-value
p_value <- sum(sample_means > observed_average_ops) / num_samples
# Print p-value
cat("p-value:", p_value, "\n")
mean_ops <- mean(sample_means)
sd_ops <- sd(sample_means)
# Plot histogram of OPS values with additional annotations
hist_data <- ggplot(data = data.frame(sample_means), aes(x = sample_means)) +
geom_histogram(binwidth = 0.05, color = "black", fill = "blue") +
geom_vline(aes(xintercept = observed_average_ops), color = "red", linetype = "dashed", linewidth = 1)
bin_data <- ggplot_build(hist_data)$data[[1]]
max_count <- max(bin_data$count)
# Plot histogram of OPS values with additional annotations
hist_data +
annotate("text", x = observed_average_ops, y = max_count, label = "Rafael Devers OPS", vjust = -0.5, hjust = -0.1, color = "red") +
annotate("text", x = mean_ops, y = max_count, label = paste("Mean OPS:", round(mean_ops, 3)), vjust = 9, hjust = -1.4, color = "black",fontface="bold") +
annotate("text", x = mean_ops, y = max_count, label = paste("SD:", round(sd_ops, 3)), vjust = 12, hjust = -2.8, color = "black",fontface="bold") +
labs(title = "Distribution of OPS Values from Permutation Test",
x = "OPS Value",
y = "Frequency") +
theme_minimal()
Heatmap of Devers and Cole’s Fastball Run-Value
# Load necessary libraries
library(tidyverse)
# Load the dataset
lefties_vs_righties <- read.csv(file = "leftiez_vs_league.csv")
# Remove statistical outliers
filtered_data <- lefties_vs_righties %>%
filter(!between(`Pitch.MPH`, 85.0, 86.1) & `Pitch.MPH` >= 85.0)
# Bin the 'Pitch MPH' into 1.5 mph intervals and 'Movement' into 1.5 unit intervals
filtered_data <- filtered_data %>%
mutate(
velocity_bin = cut(`Pitch.MPH`, breaks = seq(floor(min(`Pitch.MPH`, na.rm = TRUE)), ceiling(max(`Pitch.MPH`, na.rm = TRUE)), by = 1.5), include.lowest = TRUE),
movement_bin = cut(`Movement`, breaks = seq(floor(min(`Movement`, na.rm = TRUE)), ceiling(max(`Movement`, na.rm = TRUE)), by = 1.5), include.lowest = TRUE)
)
# Ensure that velocity_bin and movement_bin are factors for proper ordering
filtered_data$velocity_bin <- factor(filtered_data$velocity_bin, levels = sort(unique(filtered_data$velocity_bin)))
filtered_data$movement_bin <- factor(filtered_data$movement_bin, levels = sort(unique(filtered_data$movement_bin)))
# Identify the bin that Gerrit Cole falls into
gerrit_cole_row <- filtered_data %>%
filter(grepl("Cole, Gerrit", Player))
gerrit_cole_velocity_bin <- gerrit_cole_row$velocity_bin
gerrit_cole_movement_bin <- gerrit_cole_row$movement_bin
# Calculate the weighted average run value for each bin
combined_run_values <- filtered_data %>%
group_by(velocity_bin, movement_bin) %>%
summarise(weighted_avg_run_value = weighted.mean(`Run.Value`, `Pitches`, na.rm = TRUE))
# Merge weighted average run values back into the filtered_data
filtered_data <- filtered_data %>%
left_join(combined_run_values, by = c("velocity_bin", "movement_bin"))
# Custom color scale function
custom_scale_fill <- function(values, low, mid, high, midpoint, limits) {
scale_fill_gradient2(
low = low, mid = mid, high = high, midpoint = midpoint, limits = limits,
oob = scales::squish
)
}
# Plot the data using geom_tile with updated color gradient and highlight Gerrit Cole's bin
ggplot(filtered_data, aes(x = velocity_bin, y = movement_bin, fill = weighted_avg_run_value)) +
geom_tile(color = "white") +
custom_scale_fill(values = filtered_data$weighted_avg_run_value, low = "darkblue", mid = "lavender", high = "darkred", midpoint = 0, limits = c(-10, 10)) +
labs(title = "Pitch Velocity vs. Movement",
x = "Velocity (mph)",
y = "Movement",
fill = "Weighted Avg Run Value") +
theme_minimal() +
theme(axis.text.x = element_text(angle = 90, hjust = 1)) +
geom_rect(aes(xmin = as.numeric(gerrit_cole_velocity_bin) - 0.5, xmax = as.numeric(gerrit_cole_velocity_bin) + 0.5,
ymin = as.numeric(gerrit_cole_movement_bin) - 0.5, ymax = as.numeric(gerrit_cole_movement_bin) + 0.5),
color = "yellow", fill = NA, size = 0.5)
Heatmap of Devers and Cole’s Chageup Run-Value
library(ggplot2)
library(dplyr)
# Load the data
data <- read.csv("Lefties_vs._RHP_Changeups.csv")
# Clean and prepare the data
data <- data %>%
select(`Pitch..MPH.`, `Movement.Toward.Away.from.Batter..in.`, `Batter.Run.Value`) %>%
rename(velocity = `Pitch..MPH.`,
movement = `Movement.Toward.Away.from.Batter..in.`,
run_value = `Batter.Run.Value`
) %>%
mutate(
velocity = as.numeric(velocity),
movement = as.numeric(gsub("[^0-9.-]", "", movement)),
run_value = as.numeric(run_value)
) %>%
mutate(
velocity_bin = cut(velocity, breaks = seq(floor(min(velocity, na.rm = TRUE)), ceiling(max(velocity, na.rm = TRUE)), by = 1), include.lowest = TRUE),
movement_bin = cut(movement, breaks = seq(floor(min(movement, na.rm = TRUE)), ceiling(max(movement, na.rm = TRUE)), by = 1), include.lowest = TRUE),
run_value_category = case_when(
run_value < -10 ~ "Low",
run_value >= -10 & run_value <= 10 ~ "Medium",
run_value > 10 ~ "High"
)
)
# Create the plot
ggplot(data, aes(x = velocity_bin, y = movement_bin, fill = run_value)) +
geom_tile(color = "white") +
scale_fill_gradient2(
low = "darkblue",
mid = "lavender",
high = "red",
midpoint = 0, # Center the gradient around zero
limits = c(-5, 5), # Adjust limits for the gradient
breaks = seq(-5, 5, by = 1), # Custom breaks for the legend
labels = seq(-5, 5, by = 1) # Custom labels for the legend
) +
scale_x_discrete(breaks = function(x) x[seq(1, length(x), by = 2)]) + # Show every other x-axis label
labs(
title = "Velocity vs Movement with Run Value",
x = "Velocity (MPH)",
y = "Movement (in)",
fill = "Run Value"
) +
theme_minimal() +
theme(
axis.text.x = element_text(angle = 45, hjust = 1),
axis.title.x = element_text(size = 12, face = "bold"),
axis.title.y = element_text(size = 12, face = "bold"),
plot.title = element_text(size = 14, face = "bold", hjust = 0.5)
)